The magic of recommender systems
Recommendation systems explained
One powerful way to boost sales, customer satisfaction and customer loyalty is to use recommendation systems. Such a system enables you to draw up user profiles based on their behavior and to predict which products are most relevant to such profiles. A user profile specifies the properties of how much interest a customer has in a particular product.
Various websites report that a user spends less than 10 minutes on an e-commerce website. This means that when a user views each product for 10 seconds, he/she has viewed 60 products by the time of leaving the website. This is very often a fraction of the range of products on the website. There is a small chance that the customer will come across the product he/she was looking for. And that is only in case the customer knows what he/she is looking for.
Mode of action
Machine learning offers a wide range of algorithms for building recommendation systems. The recommendations are calculated based on information extracted from :
- user interaction (and transaction) data collected via the website,
- available product item data.
There are many techniques to set up a recommender system. We discuss the two most frequently applied
Collaborative filtering
This technique is still one of the simplest methods and takes into account all interactions of all users. Collaborative filtering calculates relationships between users or product items and makes recommendations with a high level of trust.
User-based collaborative filtering
The idea behind user-based collaborative filtering is to find users with the same taste as a certain target user. For example, if Julie and Sophie bought the same dresses in the past, we consider these two to be similar users and we can use the shopping history of Julie to predict Sophie’s yet unknown shopping behavior. For example, if Julie bought a red dress and a blue dress, and Sophie also bought the red dress, then the blue dress will be a logic recommendation for Sophie.
Item or content-based collaborative filtering
The interrelationships between items are calculated based on historical purchases. For example, Julie, Sophie and many other customers both bought the red dress and the blue dress. We can therefore deduce that the blue dress and the red dress must be similar and therefore we construct a neighborhood of similar items. If Caroline, buys one of the dresses in this neighbourhood (e.g. the red dress), it would be relevant to recommend other similar items to her, (such as the blue dress) to Caroline.
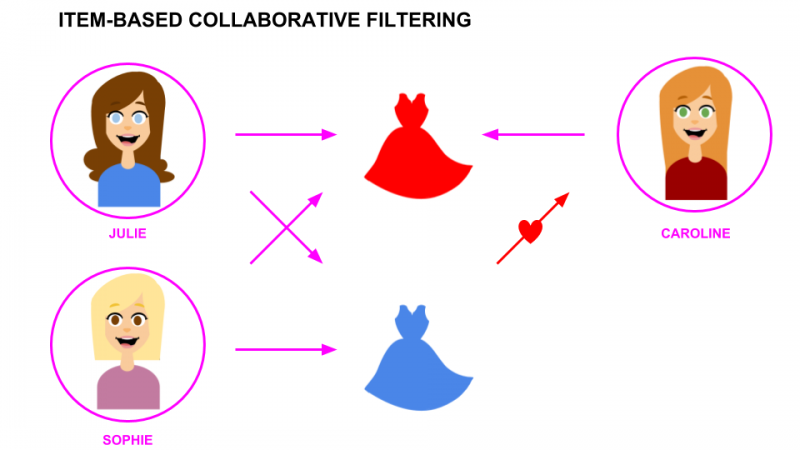
ML2Grow’s technology
Many of the recommender algorithms that are being offered in big software packages are implementing basic technology which has been developed between 2005 and 2010. At ML2Grow we make use of the latest proven advances in machine learning such as deep auto-encoders which offer a much better performance and accuracy. In addition, we customize the solution completely to match your company’s market positions, offering you an even higher return on your investment.
Takeaway
To better inform your customers about your product offering, adverts and offers are very important. Through printed media, online ads and newsletters you can inform the customer, but which products deserve extra attention? The optimal selection of products maximizes the amount of sales but a personalized selection is needed since every customer is unique. Recommender systems made by Netflix, Amazon and Facebook show the enormous power of this personalized approach.
Through advanced machine learning techniques, ML2Grow can make this selection for you. Based on the historical sales data of all customers, our recommendation engine calculates the most effective selection of products to suggest for each customer. This selection can then be integrated into existing business processes. This opens up a range of possibilities to interact with your customers in a personal way. Personalized messages, newsletters, promotions, website views, you name it.
More info? Do contact us.