Qualitative Data is what fuels AI
Quantity and quality
Artificial intelligence (AI) is revolutionising our lives and work, and data is at the heart of this transformation. From self-driving cars to personalised medicine, AI enables new capabilities and improves existing ones. However, every AI application uses some kind of data to train and power the underlying algorithms. In this article, we explore what we expect of data in AI and why it is so essential.
First, let us talk about a common misconception about using AI in a small business. You don’t need a massive volume of data to create an impact with AI.
Any AI model requires data to be modelled, but not every use-case requires large amounts of data. Specialised techniques exist that are able to extract business value from small data collections.
Now that is out of the way, let’s define what we mean by ‘data’ in this context. Data refers to anything that can be processed and analysed by a computer. This can include text, images, audio, and video, among other formats. In AI, data is used to train algorithms that recognise patterns, make predictions, and take action. In other words, AI transforms data into information.
Yes, in almost every setting, more relevant and non-redundant data allows for AI algorithms to perform better. This is because, with more data, an AI can more confidently separate spurious details from general truths that are present in the dataset. In other words, more data will allow an AI system to generalise ‘better’. For some tasks, for example, image recognition, AI systems require thousands or even millions of images of different objects to be trained from scratch. However, we should not falsely generalise this data quantity requirement to all business cases. Indeed, AI systems are also providing value in situations where only a handful of data points are available, such as optimising design parameters in various engineering use-cases.
So quantity is not always needed, but quality definitely is. The data to train AI systems must be diverse, accurate, and relevant. For example, suppose an AI system is trained to recognise objects in an image. In that case, it needs to be shown images of different objects from different angles and under different lighting conditions. If the data is not diverse, the AI system will struggle to generalise and will not be able to recognise objects in different contexts.
Another critical aspect of data is its relevance. The data to train an AI system must be relevant to the task. For example, if an AI system is trained to recognise objects in an image, it should be shown images of objects, not landscapes. Relevant data helps the AI system to focus on the task at hand and improves its performance.
Finally, data needs to be accurate. The data used to train an AI system should be as free of errors and biases as possible. Some techniques are prone to being sensitive to outliers or inaccurate data. Although a good data scientist will spot this and take care of the problem, it introduces extra challenges and risks.
There are various AI techniques and use-cases that together provide value using only limited data: such as data efficient machine learning, transfer learning, data augmentation and synthetic data generation.
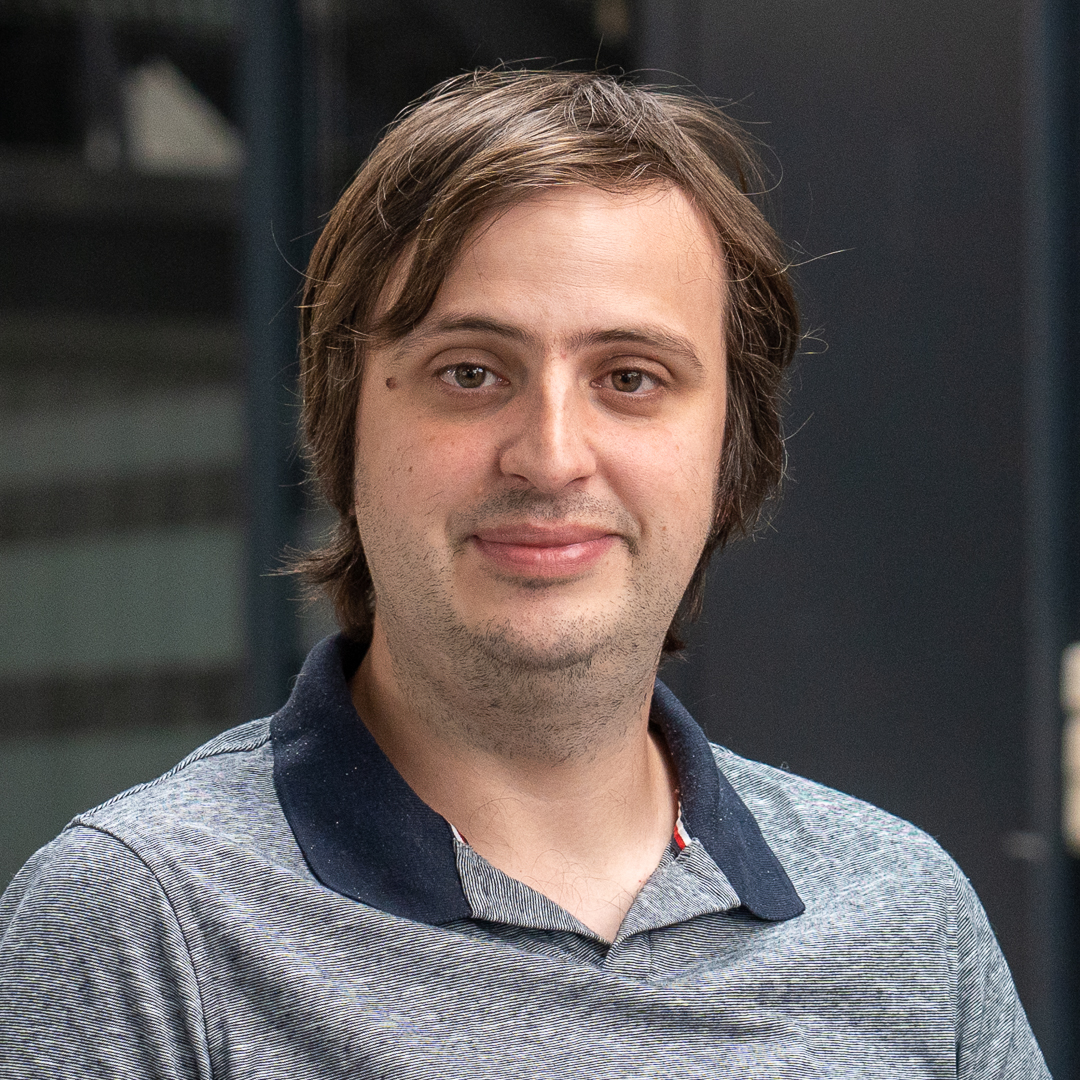
AI for small businesses
As we said, with recent advancements in AI technology, it is now possible for SMEs to gain insights from small data sets. For example, an SME with small data on customer interactions can still use AI to gain insights into customer behaviour and preferences.
One of the most potent ways SMEs can use AI is through predictive analytics. Predictive analytics uses historical data and machine learning algorithms to make predictive events. For example, a retail company could use predictive analytics to forecast demand for a product, which would help them make better decisions about inventory management.
Another way that SMEs can use AI is through natural language processing (NLP). NLP is a branch of AI that deals with understanding and generating human language. SMEs can use NLP to automate customer service, for example, by creating chatbots that can respond to customer inquiries. NLP can also be used to analyse social media data and gain insights into customer sentiment. Recently, NLP is also used frequently to automate information extraction from documents, e.g. to assist in the invoicing workflows. In some non-critical cases, it can be combined with Robotic process automation (RPA) to achieve an (almost) autonomous process.
We have already written about NLP in the past, and you can find the blog post at the end of this post.
One of the biggest challenges that SMEs face when it comes to using AI is the cost. AI technology can be expensive, and many SMEs may not have the resources to invest in it. However, many solutions start from open-source AI models that are free to be re-used in a commercial context. In such cases, a limited integration and customisation step are all that is needed to achieve a working and robust solution.
ML2Grow is a vendor-neutral solution provider, meaning we integrate with any existing cloud platform or other systems. We offer end-to-end solutions, from ideation to hosting the perfect solution on our computing infrastructure, without introducing a vendor lock-in.
Please look at our MLOps page at how we can provide a complete end-to-end solution tailored to your need.
You know your business; we know technology. Together, we can accelerate value creation for your business through innovation. Bringing your ideas into reality is not easy, but we are here to help and guide you with every step. Most SMEs have limited AI knowledge or don’t have the time or budget to experiment with AI. Our experts will guide you from an idea to a profitable, impactful, integrated AI solution.
AI roadmap
Due to old prejudices, many companies miss opportunities to optimise their business processes through AI.
Although the adoption threshold for AI is now drastically lower on several fronts in recent years, many decision-makers still think that AI is too complex, requires too significant investments and is only suitable for large companies.
For companies that appreciate the value, the road to successful adoption seems long, arduous, and full of obstacles.
The big problem is that these organisations often need a clear plan for the management to get started.
Our team of experts has already prepared a straightforward guide that will make the road to the top much smoother.
Download now and discover all the pitfalls and opportunities when implementing AI technology in your organisation.