Unlock intelligent planning with AI-driven forecasting
Forecasting
Businesses conduct forecasts in all areas of an organisation. Finance teams generate revenue forecasts reflecting new scenarios and opportunities so executives can maximise value. Employees across the supply chain and human resources need to accurately predict future workforce needs to optimise inventory or staffing levels. Marketing teams leverage forecasting to allocate promotional spending where it will pay off the most.
Regardless of the department, the traditional forecasting process remains the same: a lengthy exercise conducted by some specialists who try to glean insights from what’s often a tiny slice of data and gut feeling. By the time a forecast is handed off to a decision-maker, valuable new data may have become available, sending these specialists back to the drawing board to re-forecast a scenario.
This manual, labour-intensive process is not flexible and can often delay critical decisions. Luckily, AI can help solve these and more forecasting challenges. AI-driven forecasting uses historical data to extract specific patterns in the data to create a statistical model that could predict the future. The more comprehensive the data we have, the more accurate the forecasts can be. Forecasting involves developing models to understand the data to understand the underlying causes. Analysis can provide the “why” behind the outcomes you are seeing. Forecasting then takes the next step of what to do with that knowledge and the predictable extrapolations of what might happen in the future.
AI-driven forecasting could also unlock intelligent planning. Critical business decision-makers can independently leverage accurate, future-facing insights by AI and data.
Intelligent planning empowers planners and decision-makers to model multiple scenarios and shape future business outcomes—with a complete understanding of commercial, operational, and financial implications of their decisions across any line of business. In 70% of predictions, ML or statistical forecasts are proven to deliver the same or greater accuracy than an expert.
For example, if a retailer wants to fine-tune its stock level on every product, they sell in his store, AI- and ML-based forecasting would be ideal. The company could potentially predict demand for every article for the coming days & months by incorporating both historical data and data attributed to every unique theme. What’s more, by adding specific external data such as weather patterns, the AI-aided forecasting can get specific enough to help predict, let’s say, an uptick in chocolate milk volume being sold because rain & cold weather are expected in the coming days.
Compared to traditional statistical forecasting, ML forecasting capabilities can deliver more accurate results. A McKinsey study found that the improved accuracy leads to a 65% reduction in lost sales due to inventory out-of-stock situations and warehousing costs decreasing around 10 to 40%. ML-based forecasts can incorporate a wide range of historical (like promotions, packaging, …) and external data (weather, public holidays, vegetable seasons like ‘spinach’ season will boost sales volumes of cream) that helps deliver a finely tuned prediction. In contrast, traditional forecasting typically leverages just a slice of internal historical data, which can yield inaccurate predictions.
Moreover, AI and ML can learn from these new datasets over time, providing business users with highly accurate predictions and explanations to help hone their decisions.
In particular, business forecasting is generally divided into two main categories:
A McKinsey study found that the improved accuracy leads to a 65% reduction in lost sales due to inventory out-of-stock situations and warehousing costs decreasing around 10 to 40%.

Demand Forecasting
It’s the process of predicting what the volume of demand of specific product articles will be in the next days-months-years by discovering patterns between the sales data (sales orders, shipping data,…) and internal data like promotions, packaging size, new articles;… from your ERP system and external data like seasonality, public holidays, weather your ERP system so we can these patterns generalise them into the future. However, only recurring effects can only be taken into effect. For new products that lack historical data, demand forecasting can identify clusters of prior products with similar characteristics and lifecycle curves and use those datasets as a substitute to make predictions.
Demand forecasting helps the business make better-informed supply decisions that estimate the total sales and revenue for a future period.
Through demand forecasting, businesses can optimise inventory by predicting future sales from analysing historical sales data to make informed business decisions about everything from inventory planning, and warehousing needs to running flash sales and meeting customer expectations.
The hardest part is transforming all these data to be correlated to each other correctly. The key is to use time as a reference base in demand forecasting to distil the necessary patterns.
For your organisation, there’s no machine-learning expertise required. We develop modular blocks that are easy to interface with any application. The results are Ultra-granular forecasts per product, day and store or fulfilment channel.
You don’t have to develop complex models; get the training data and label the data yourself. Everything will be tailored-made to your needs and explained by our team. Our models can reach an accuracy of up to ≥90% by analysing three basic patterns: demand patterns (seasonality trends,…), business decisions (promotions and price changes, product changes, …) and external factors (holidays, weather, local events,…).
Benefits
Preparing your budget
Demand forecasting helps reduce risks and make efficient financial decisions that impact profit margins, cash flow, allocation of resources, opportunities for expansion, inventory accounting, operating costs, staffing, and overall spending. All strategic and operational plans are formulated around forecasting demand.
Planning and scheduling production
Demand forecasting lets you provide the products your customers want when they want them. Forecasting demand requires that order fulfilment is synced up with your marketing before launching. Nothing kills progress (or your reputation) faster than being sold out for weeks. Proper demand forecasting and inventory control can help ensure a business doesn’t buy insufficient or excessive inventory.
Storing inventory
Demand forecasting can help you spend less money on inventory purchase orders and warehousing, as the more inventory you carry, the more expensive it is to store. Good inventory management involves having enough product on hand but not too much. Closely tracking inventory levels lets you easily restock and forecast inventory over time.
Extension of demand forecasting into Financial forecasting
Embracing uncertainty means planning many plausible future scenarios and recovery paths – building the agility to navigate today’s unknown. AI allows you to create new and more insightful scenario-related models to deal with external forces and support strategic decisions. It becomes your source of truth, processing massive amounts of data quickly and calibrating scenarios in near-real-time. Humans can then shift away from feeding the data to take strategic action as AI offers continuous insight to guide them. That packs a powerful punch in terms of symbolic value and creates a compelling reason to assess how you can bring AI into your scenario planning process now.
Churn forecasting
Did you know that acquiring a new customer costs five times more than keeping an existing one?
Customer churn can manifest differently for different businesses. Still, it can be generally defined as what happens when a customer unsubscribes from your service, ceases to purchase from you or stops engaging with your brand.
But how do you deal with customers who drop out or suddenly stop ordering? This is a complex problem: customers are diverse, interact with your company differently, and have reasons to stop the cooperation. Detecting customers who are about to leave but who have not yet finalised their decision is the purpose of churn prediction.
Too many companies fail to address customer churn adequately. While usually no expense is spared to find and attract new customers, the same seldom applies to identifying customers ready to join your competitor. But how exactly can you focus your efforts on the right customer?
We see more than ever that general churn prediction algorithms offered out-of-the-box in data platforms do more harm than good. They were designed to work in a general way and fail to understand the specifics of your unique company-client relationship.
Calculating an accurate churn rate is complex: it involves a lot of different formulas, effort, interpretation, and to be honest, it’s quite a mess to do it the classical spreadsheet or business intelligence way. However, there is a more precise, accurate way to produce this information. As you may have guessed, the answer lies in data science and machine learning.
Machine Learning helps pick up purchasing behaviour patterns by sifting through enormous amounts of data and identifying common attributes of customer behaviour. This translates into identifying what kinds of customer action most often leads to churn, even if that action is something small or not easily measurable. The significance of predicting customer churn is not just the data provided, though that in itself is pretty remarkable. The true importance lies in what marketeers and salespeople can then do with that information, which is to understand your customers better and reach out to them according to where they are in the customer lifecycle. It turns something informational or analytical and takes it a step further to make it actionable. Identifying which customers are at risk of churning helps you know when you need to send them an offer to win them back. Predicting when they are most likely to churn gets you the information you need.
For example, the following figure is taken from a real use-case where classical and general AI models fail to be truly intelligent systems.
The graph represents the quantities of different items a specific customer bought over time, and the other lines represent different products. Do you notice something special? Indeed, whenever the customer purchased the orange product more, he ordered less of the blue product and vice-versa. In this setting, a classical out-of-the-box system would constantly predict individual product churn on one of the two items. In reality, however, the customer had been loyal for years and simply required more of the orange product when it was warm and more of the blue product when it was cold outside.
This is where our custom made machine learning models come in to help make predictions. A specific churn risk is calculated individually per customer based on the knowledge and insights extracted from all your customer interactions.
This approach gives companies a deeper and more accurate understanding of each customer’s behaviour. Also, it gives you a much better chance to promote enduring customer relationships. Covered with this knowledge, companies can use personalized campaigns for customers at the right time to encourage loyalty and customer engagement.
ML2Grow has developed a method to accurately predict customer churn in which our unique approach for individual churn cut-off calculation is fundamental. This method has been tested and validated as an accurate and effective method.
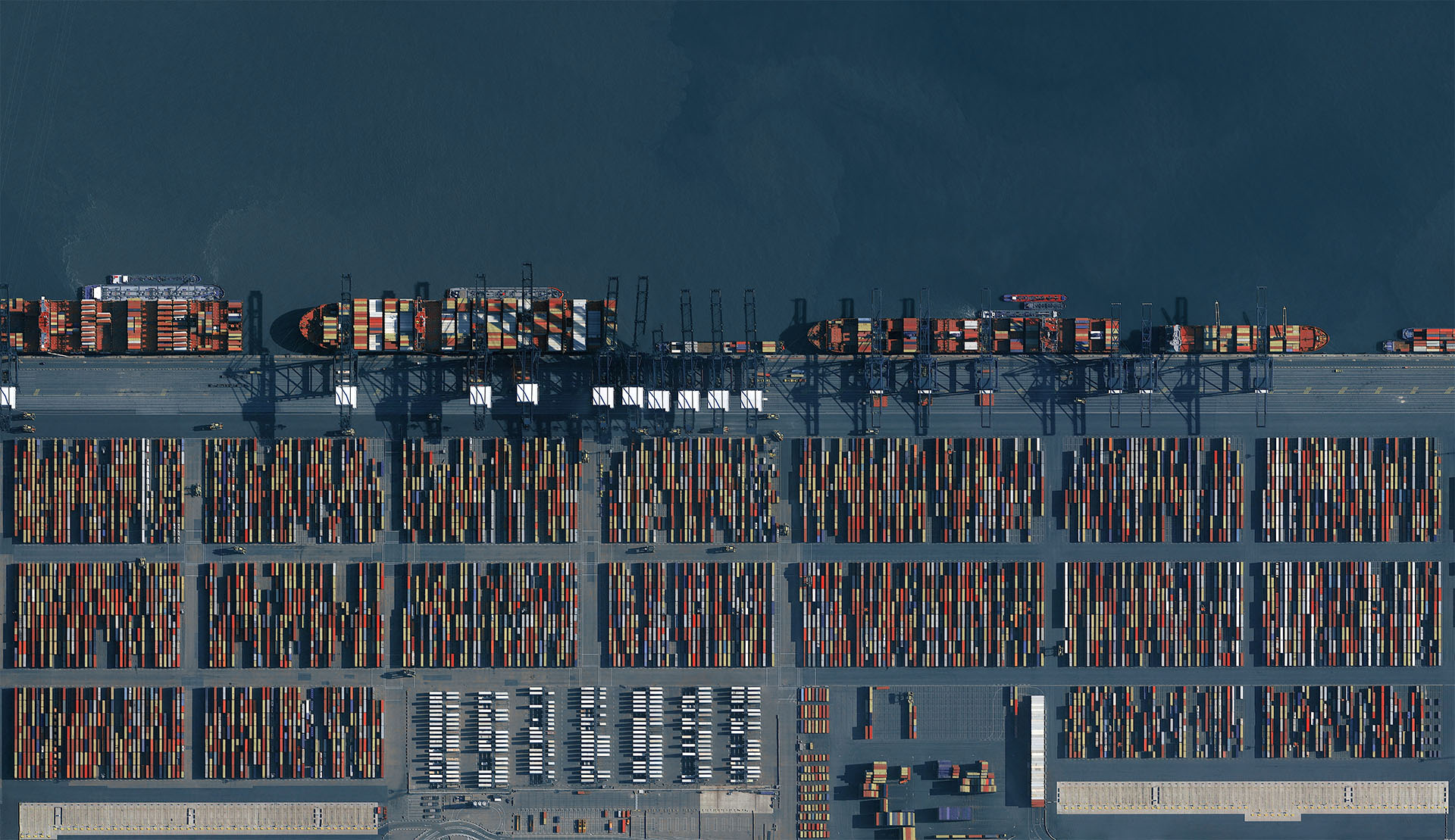